Renewable Energy Generation Needs Critical Data
INTRODUCTION
One of the main actions to fight climate change is the increasing use of renewable energy leading to the rapid growth of renewable energy deployments on grids worldwide. It has profoundly changed the electric power value chain and bringing new operational challenges. Distribute energy resources (DERs) located across wide geographic areas and two-way power flow from inverter-based devices, along with the risk of extreme weather conditions that can disrupt power resources, combine to form complex problems that must be solved in time and acted upon thousands of times each day to ensure uninterrupted, reliable power.
High-quality and reliable renewable energy resource data and other geographic information system data are necessary for the shift to a clean energy economy that focuses on local resources, improves resiliency, increases employments, and encourages the self-sufficiency of energy. These data are crucial for making informed decisions—ranging from policy and investment decisions to reliable power sector planning. [rml_read_more]
Decisions that are data-driven reflect the appropriate ambition to maximize cost-effectiveness and enable the successful implementation of renewable energy investments. Various considerations are essential in planning for data-driven decision-making. For instance, data can vary in type and quality, be expensive to obtain, and require specific skills and resources to process and interpret.
In this article, we will discuss data requirements for making various renewable energy decisions and discusses the tools and analyses necessary to transform these data into recommendations for decision-makers.
NEW CHALLENGES TO RENEWABLE ENERGY
- Meeting the Requirements of Availability and Reliability– One of the biggest concerns in the field of renewable energy is their availability depends on natural resources that are uncontrollable by humans. For example, solar-powered electricity is generated only when sunshine is available and turns off at night; wind energy also depends on the availability of wind, so if the wind speed is deficient, the turbine will not turn, and this result in zero power flow to the grid. Another is reliability, fewer generating sources can’t meet the peak demand. It has been found in a study that there is a narrow reserve margin during peak loads in the case of renewable energy generation. That narrow margin offered little room for error and heightened the need for accurate, data-driven weather and market forecasting to inform operational decisions.
- Markets and Profitability- Here, the strategic use of data enables energy providers to adjust operations in real-time to deal with the highly dynamic energy, based on market conditions and keep their generating assets in the profit.
For instance- the grid operators have to manage Security Constrained Economic Dispatch twelve times an hour, mostly an energy auction that ensures that the lowest-cost resources are dispatched. This model will work with more efficiency if it uses data and redesigns market-based upon information, it will provide a handful of zones with hundreds of nodes where action can be taken.
- Achieving Real-time Situational Awareness of Distributed Energy Resources– The growing installation of distributed generating assets includes not only wind farms but also thousands of small-scale rooftop solar installations. Real-time data from these distributed assets prove indispensable in helping asset managers and grid operators achieve real-time situational awareness and improve outage prevention and response strategies.
THE AGE OF RENEWABLE ENERGY
The energy business is functioning in a shifting world where renewables and DERs are becoming an increasing part of the energy generation mix. This decarbonization of the grid has been a key driver of network change in recent years.
In 2017, renewables contributed almost 50% of the growth in global power generation and now account for 8.4% of global electricity generation (excluding hydro), with growth rates rising year-on-year. In the UK, for example, renewable generation has increased from 5% to 30% in the last ten years with over 25% is already connected to the grid at the distribution level[1].
In Africa, there is a strong push towards renewable energy sources to increase generation capacity and the pace at which clean and affordable energy access is rolled out. The increasing cost-competitiveness of wind and solar power are making these technologies a very attractive option for African countries looking to achieve rapid and cost-effective expansion of electricity access for their growing populations[1].
Renewables also offer greater flexibility with micro-grids providing rapid local solutions that can be connected to the main grid at a later date. This pace of change is only set to accelerate as governments pursue clean growth strategies, energy markets become decentralized and electric vehicles become increasingly common.
Renewable technologies are becoming accessible because of cost reductions as innovative materials and engineering solutions are applied to solar photovoltaics and wind power. These enabling factors are paying off with impressive results for renewable energy.
For example, the Paris-based International Energy Agency (IEA) expects renewable electricity generation worldwide to rise by 920 gigawatts (GW) by 2022, an increase of 43% from 2017. And for the first time, additions of solar photovoltaic (PV) technology in 2016 rose faster than those of any other fuel.
OPERATIONAL CHALLENGES TO RENEWABLES
For operators and fleet managers, the rise of alternating generating resources has appeared to be tricky for traditional generating resources like coal and nuclear. Those plants were designed and built to run continuously; no one expected them to ramp up and down any more often than for a refueling or maintenance outage. Now, however, many baseload units cycle regularly as alternating resources enter and exit the market.
For operators, the shifts in renewable generation can be challenging without accurate data and forecasting capabilities. For example, almost a decade ago, an Energy company was shutting down gas and coal generating resources to establish hundreds of MW of wind energy farm during favorable conditions. But when wind speeds are high, the wind turbines would “clutch” and stop producing power. The sudden loss of more than 1 GW of energy forced to reopen the turned-down coal and gas plants compensate energy demand. The utility always needs better data to forecast resource availability with all its terms and condition so that they can be managed optimally.
If we talk about a distributed generation from solar, operators also must manage two-way power flows, that can be achieved by VPPs. Virtual power plants made it possible by aggregating large amounts of distributed rooftop solar capacity by rapidly converting a consumer into a power producer and vice versa, depending on weather conditions and time.
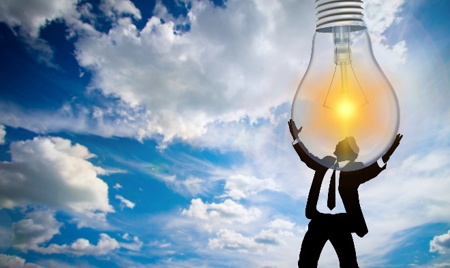
UTILIZING DATA
The amount of data is growing exponentially, helping the power plant operators as well as fleet and grid managers to make critical decisions about the operation and marketing. A single renewable farm may have more than 1000 generators units. Operators need to access their status every second about their voltage and current information, and millions of smart meters are required to report consumption.
The increasing amount of renewable energy capacity and the expanding deployment of distributed, customer-owned generation mean that more data and faster responses will be needed to make operational decisions to balance supply and demand.
Meeting the challenge to maintain availability and reliability, while integrating increasing amounts of renewable energy, requires treating data as a critical asset. The importance of data is changing the role of IT departments within utilities as they are responsible for turning data into a reliable and available enterprise asset that can inform decision-making for effective integration of renewable power.
OTHER ROLES OF DATA IN THE ENERGY SECTOR
Operations, Planning and modeling, and Forecasting are the three fronts where data can be utilized effectively:
- Operations-Real-time tools allow operators to have more dynamic control over the market. Monitoring tools deployed in Power farms updates real-time conditions about the transmission system, operating limits, power generation, consumption, etc. Occasional assessment may be disadvantageous, as it lacks more frequent insights into grid dynamics led operators to be too cautious.
- Planning and Modeling- As the global economy becomes increasingly electrified, not just in the power sector but across transportation, heating, and cooling as well, electricity demand will rise by 62%, leading to almost a tripling of capacity by 2050 and more than $13 trillion of investment, of which wind will take $5.3 trillion and solar $4.2 trillion. In addition to the spending on new generating plants, $840 billion will go to batteries and $11.4 trillion to grid expansion[3].
According to Outlook, new wind and solar farms, often with battery storage, will be able to produce power more cheaply without subsidies than already-built coal and gas plants almost everywhere in the world by 2030. As a result, the role of coal in the global power mix will fall from 37% today to just 12% by 2050 while oil as a power-generating source will be virtually eliminated, as wind and solar grow from 7% of the generation today to 48% by 2050[3]. The contributions of hydro, natural gas, and nuclear remain roughly level on a percentage basis.
A report suggests that by 2031 there will be between 15 and 30 GW of solar PV added to its system, around a 3,000% increase over 2017 in Texas.
- Forecasting- The growing numbers of renewable resources might solve the problem of shortage during peak demands. Prediction of power demand plays an essential role in the electric industry, as it provides the basis for making decisions in power system planning and operation. A great variety of methods for predicting electricity demand are being used by electrical
companies, which are applicable to short-term, medium-term or long-term forecasting. But changing the electrical demand arises from complex interactions between personal, corporative, and socio-economic factors. In such a dynamic environment ordinary forecasting techniques are not sufficient, and more sophisticated methods are needed.
PREDICTIVE ANALYSIS SUPPORTS PROFIT
A Sydney based Australian utility manages one-third of the energy of the country’s populous eastern seaboard. Their generating capacity grew from 300 MW in 2005 to 5,500 MW by 2012 and planned to further that amount to 9,000 MW. Even so, the utility had little actionable data to help it assess performance.
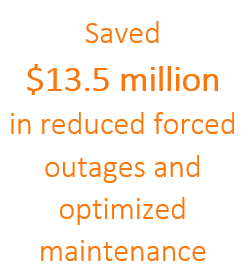
The utility started implementing the smart data infrastructure so that employees could create drag-and-drop custom visualizations of real-time data. Then, in April 2015, with an operational diagnostics center, enabled the utility company to start predictive modeling based on the data they received. They deployed anomaly detection software for advanced pattern recognition and built some 2,700 models to monitor 12 times an hour some 45,000 critical data points.
In three years, the utility has saved AUD 18.7 million ($13.5 million) in reduced forced outages and optimized maintenance. Additionally, in 2017, they caught and prevented a potentially catastrophic failure in a hydrogen-cooled stator. In this incident, system alarms started ringing and the analytics team noted anomalies in the hydrogen exit temperatures. After recalibrating the instrument, operators put it back online, but the data showed a worsening problem. The team planned an outage to investigate, and, after a partial dismantle, found that the stator was mere days from having a number of its coils catch fire. A forced outage, which was avoided thanks to predictive analytics, could have cost anywhere from AUD 50-70 million ($36 to $50 million).
DATA-EMPOWERED DECISION MAKING
At every stage of electricity generation and distribution, data supports better decision making and help optimize cost, increases assets life. The trend of renewable energy, bi-directional power flows, and DERs has attracted the focus on the need for actionable real-time data. The future belongs to those who realize that energy companies are now in the business of managing data as much as power. The most progressive understand that you can no longer do one without the other.
ARNOWA’S OFFERING IN RENEWABLE ENERGY
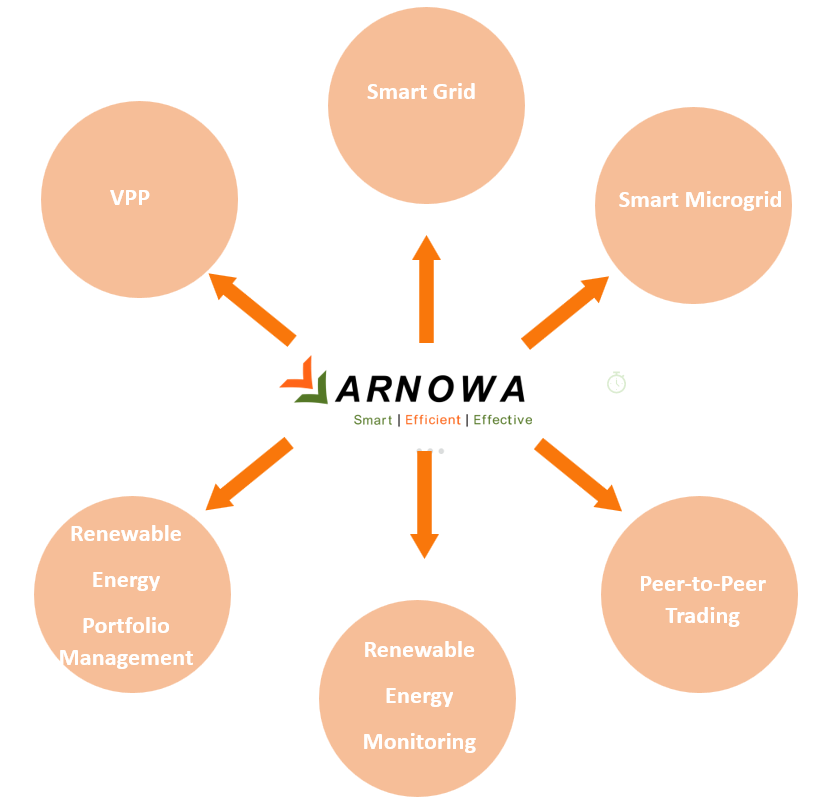
REFERENCES
[1]ESI Africa, 2019. The age of renewable energy. Link: https://www.esi-africa.com/regional-news/international/the-age-of-renewable-energy/
[2]International Renewable Energy Agency (IRENA). The Age of Renewable Power: Designing national roadmaps for a successful transformation.
[3]Mike Scott, 2019. Wind And Solar Power Set To Dominate Power Mix By 2050, As Coal Continues To Decline. Link: https://www.forbes.com/sites/mikescott/2019/06/24/wind-and-solar-power-set-to-dominate-power-mix-by-2050-as-coal-continues-to-decline/#31bdb99255b6
[4]Meet A. Moradiya, 2019. The Challenges Renewable Energy Sources Face. Link: https://www.azocleantech.com/article.aspx?ArticleID=836
[5]Union of Concerned Scientist, 2017. Barriers to Renewable Energy Technologies. Link: https://www.ucsusa.org/resources/barriers-renewable-energy-technologies
[6]Electricity from Renewable Resources: Status, Prospects, and Impediments, 2010.Chapter: 4 Economics of Renewable Electricity. Link: https://www.nap.edu/read/12619/chapter/6